For my next music video, I thought it would be fun to a fine tune a stable-diffusion model with Cyberpunk 2077 images. My plan was as follows:
- collect about a thousand images from the games photomode system
- provide natural language captions for them
- and then train a stable-diffusion checkpoint on that data.
It was fairly quick to find a 1000 images from the game and with the help of BLIP and manual labor I created captions for all of them.
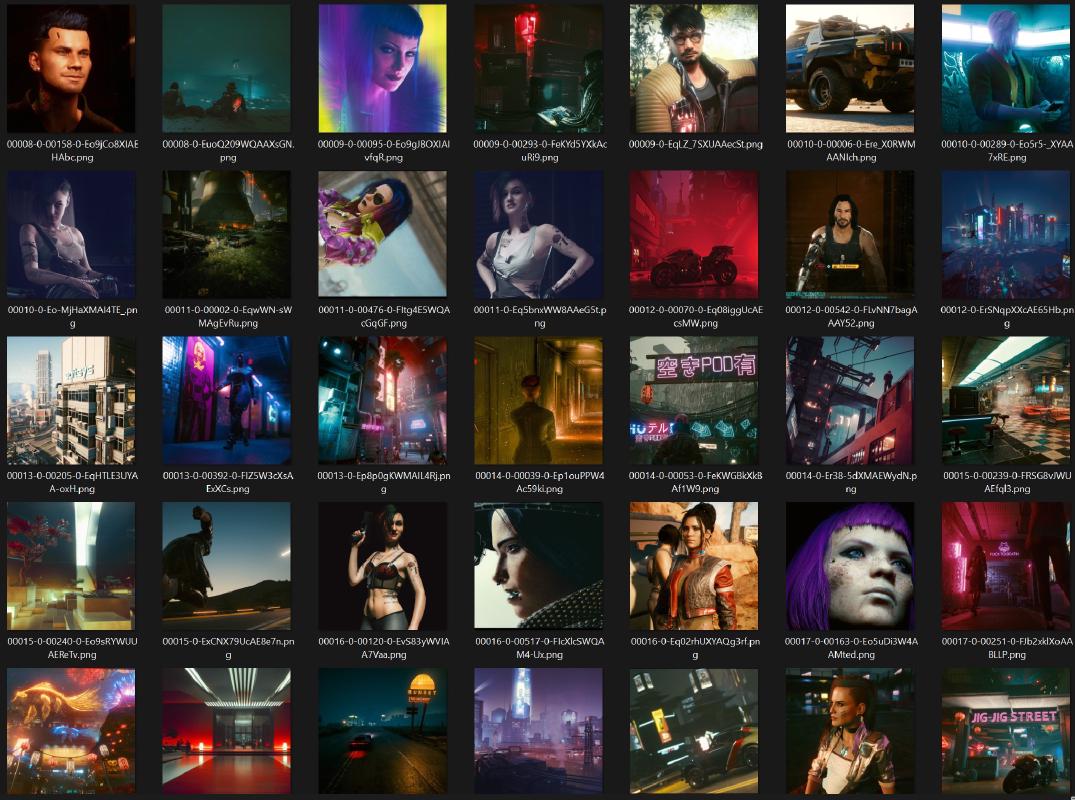
Training requires bigger GPUs than I have access to myself. Fortunately, LambdaLabs provides access to Cloud GPUs at a price that’s about 5x cheaper when comparing to GCP. Although, LambdaLabs was quite oversubscribed I was able to rent an NVidia A100 sxm4
with 40GB
of memory for $1.10/hour; this is compared to a purchase price of about $10,000.
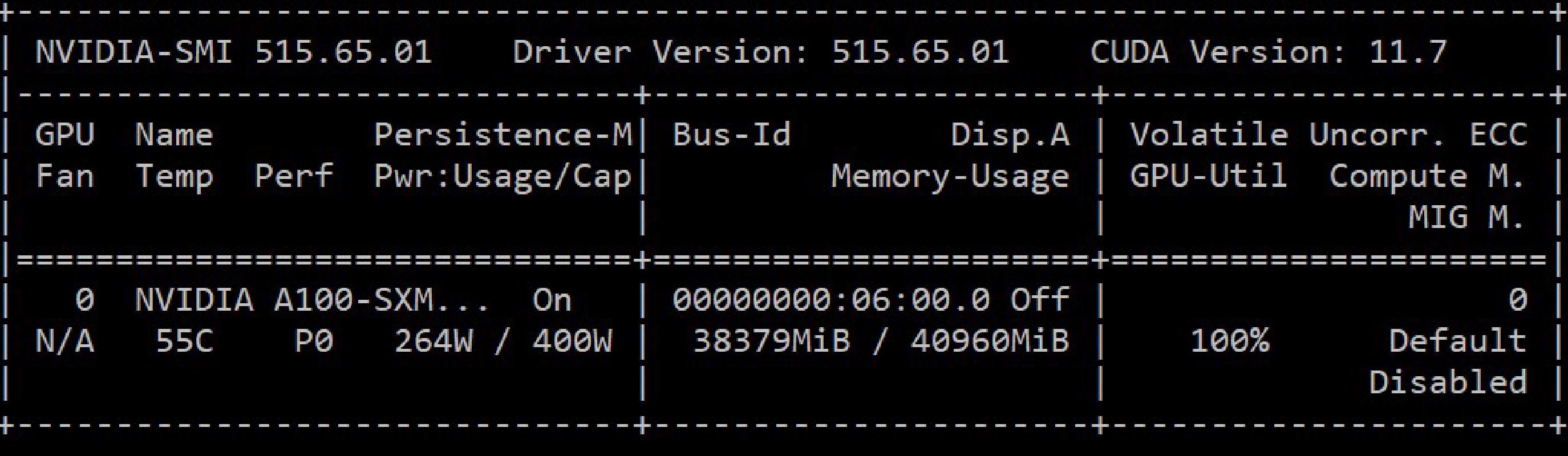
The repo from Justin Pinkey provided a great starting point for this and was super easy to use. The only changes I made were additional steps to boostrap the environment on an empty Ubuntu installation and a small tweak to the dataset loader to accept directories with image files and corresponding captions rather than downloading a dataset from Huggingface.

After training for 219 epochs which took roughly 20 hours, I ended up with about 15 checkpoints each 14GB in size; with pruning the checkpoint can be reduced to 4GB and by converting to fp16 the size can be reduced even further. Here is a test grid from the model for the simple prompt: a woman
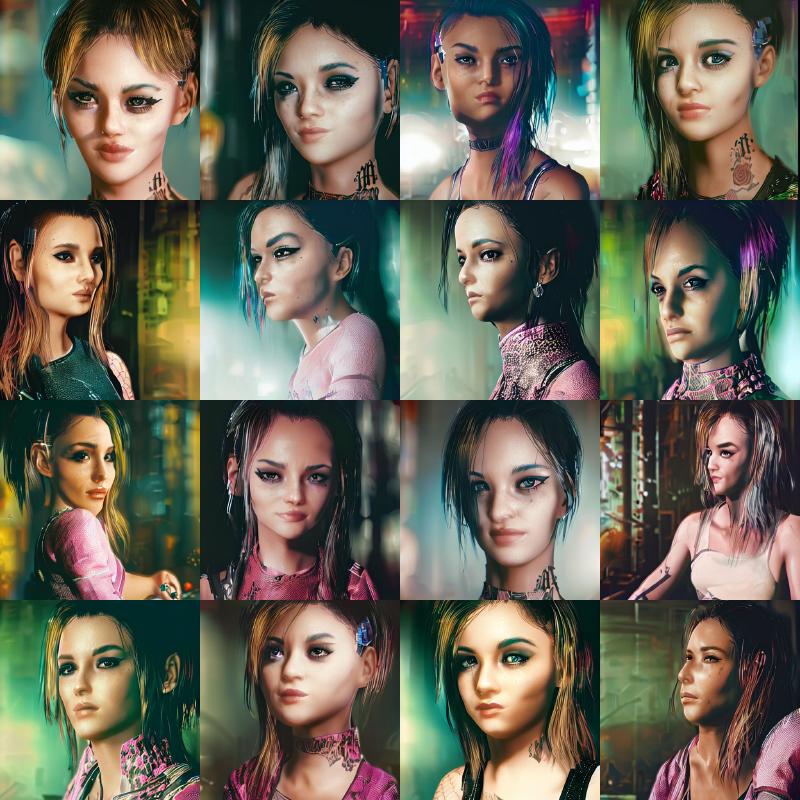
You don’t want to see the test grids for more complex prompts. They looked terrible and I’ll just show you just one example:
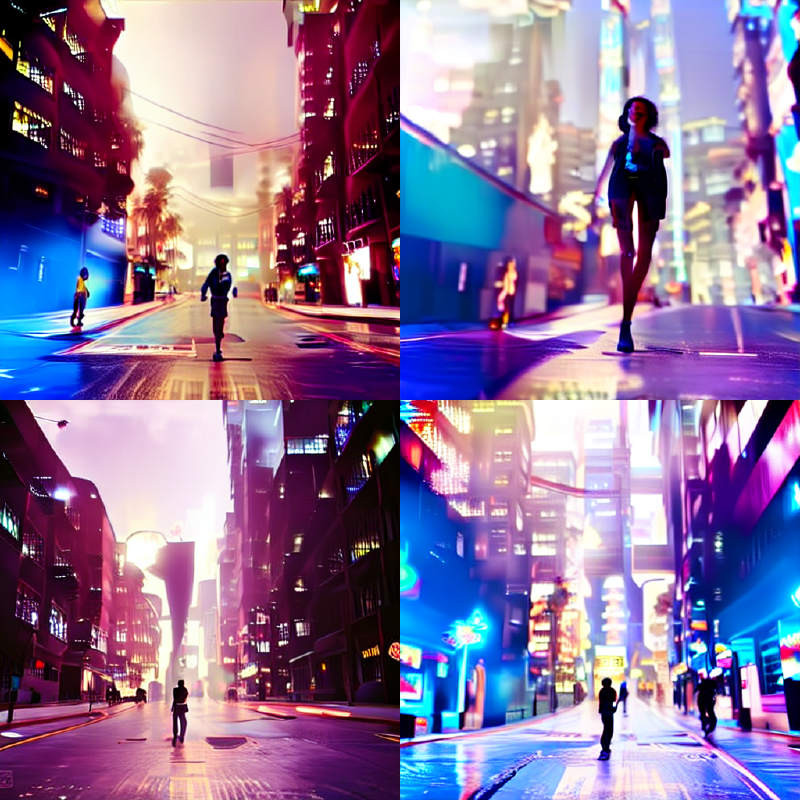
Some of the problem with training was likely that I did not have enough images and that unlike the pokemon example, the images were visually more varied while not being very diverse. By going with photomode pictures, I ended up with very pretty shots but they mostly showed a few of the game’s prominent characters, e.g. Judy or Panam. That said this experiment was relatively inexpensive.
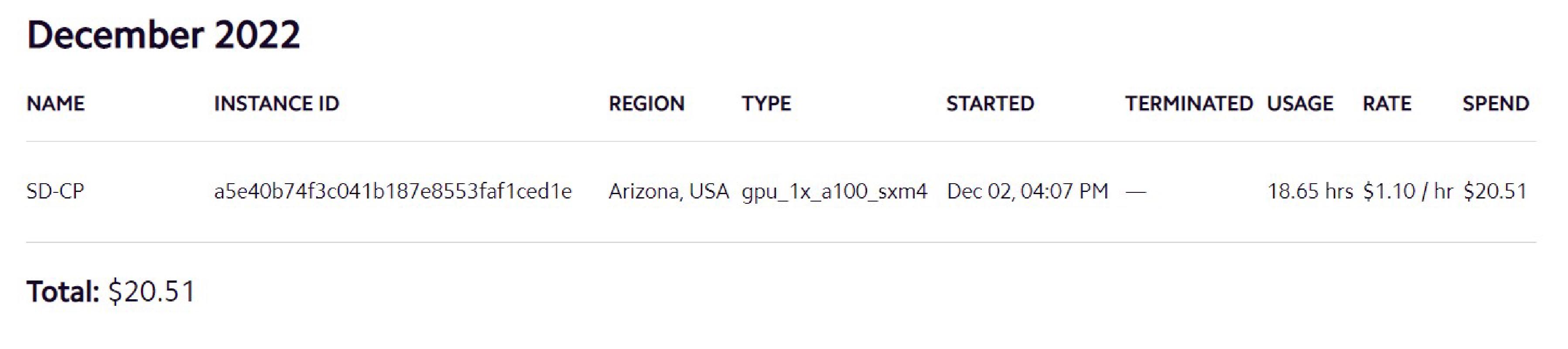
And overall, this was quite worth the experience. Onwards and upwards.